Finding Common Time
Vincent Brandon, Data Coordinator
March 3, 2021
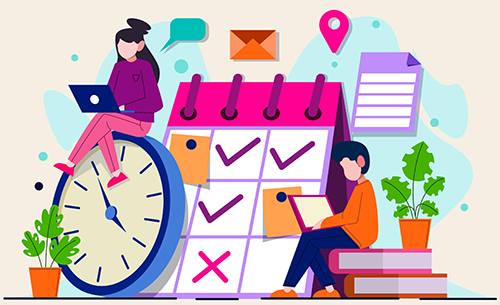
Time series analysis has become ubiquitous. From social science researchers to marketers and data engineers building intelligent QA systems, time is more important than ever. Time, though, does not standstill. Neither do people. The UDRC maintains one of the largest cross-agency longitudinal datasets about people in Utah. For UDRC to effectively build reports for our clients, it is important to note that our time-series datasets come with baggage. Time is not a date. Dates are not always what they seem, and timestamps are sometimes the fault of unassuming database managers that did not set the field correctly.
On top of that, people come and go in every set. Cohorts may only exist in part of the data space. To help manage these issues, here are a few considerations for your future data request.
What you need to be aware of before you hit submit on your request
The first caveat: Have an idea of how you expect your study cohort to change over time. We often have to use proxies for certain behavior, and it helps to document how data is acting as a stand-in for ground truth where direct observations are not available.
The second is to ask, “What design will elucidate the theoretical issues I am addressing?” You know what you are measuring. If you could start from scratch, how would you optimally design the study? Is it longitudinal, one group over a long period, or sequential? People change. Times change. Maybe it is best to look at a certain period in life in as many groups as you can—for example, all the freshmen from 2010 to 2020. The goal is to select the points in a group or individual’s lifecycle that correspond to the process you are looking at. Rather than reviewing arbitrary scales of available data, it is better first to understand if specific periods of time correspond to the process or events in question. Understanding this helps create period exclusion criteria for your request.
An example might be to exclude students who are working or only keeping those with stable employment after graduation. Diving into these details helps create censor bounds for the request. For example, students must have graduated before the last year of available wage data to ensure stable employment records.
Finally, match your request design with statistical procedures that will best exploit the data you will receive. Having the model in front of you at the time of request ensures that we will capture known correlates, establish useful controls, and structure the dataset so that you do not have to do unnecessary transformations. This preparation can also help eliminate unneeded data from the request, leading to faster turnaround times.
Now a couple of caveats. Not everyone uses the year the same way. Exact dates are not meaningful to everyone. When you request data that crosses partners, we may need to shift all dates onto a common scale. The academic to calendar transition or binning quarterly wages into tertile semesters should be transparent and meet the needs of your analysis.
We at the UDRC are here to help! These are fun conversations, and everyone generally learns something every request, even about data we stare at every day. Do not hesitate to ask where to start at udrc@utah.gov.
References
Lerner RM, Schwartz SJ, Phelps E. Problematics of Time and Timing in the Longitudinal Study of Human Development: Theoretical and Methodological Issues. Hum Dev. 2009;52(1):44-68. doi:10.1159/000189215
https://courses.lumenlearning.com/suny-lifespandevelopment/chapter/research-involving-time-spans/