Data Enrichment
Vincent Brandon, Data Coordinator
December 1, 2021
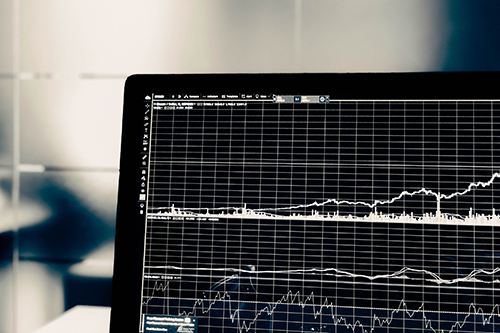
What is Data Enrichment?
Data enrichment is the process of adding related information to the source material to gain better insights and understanding. Data enrichment is done every time we do a sensible join in a database or compile lookup columns in Excel documents. For example, researchers regularly request their core data be enriched with behavioral, demographic, or geographic markers. That is not the limit, though. Raw data is scarce and often contaminated. In addition, we can add thoughtful information about the quality and reliability of data for a new class of audits.
Real-time vs. on-demand enrichment?
Real-time enrichment means that users of the data can see enrichment outcomes immediately upon viewing the source material. A report with extra columns is real-time enriched for anyone reading the report. Most enrichment is unavailable in real-time. There are near-infinite combinations of relationships of interest across datasets. Which to look at is a large part of expert analysis.
Passive enrichment means clean, linked data is available for consumption on demand. Users need to provide a query to obtain the views they desire. Passive datasets have often been preprocessed, cleaned, and keyed to values in core datasets to make joins across sources easy for end-users. The UDRCs primary passive linkage is the Master Person Index (MPI). MPI can link any personally attributed data from multiple sources without exposing PII or making end-users generate complicated joins.
How the UDRC is enriching source data for its researchers
The UDRC is implementing new real-time and passive data quality measures for its de-identified datasets. The UDRC’s users are researchers building reports. For them, we will offer two methods of screening for data quality.
Passively, we are now flagging indices for potential issues. Researchers can join their population of indices (remember indices reference possible individuals in this case, though in a roundabout way) to a set of flags continuously running in the background. Researchers can then choose to keep or remove data points based on whether or not an index has some specific flag or set of flags.
We combine these flags with a probability of a match for any of our MPI indexed data points. Every row of every table will have an independent classification score ranging from 0 to 1. The closer the probability of a match is, the more confident our models believe the row was matched correctly to a new or existing index.
Given a passive pool of flags and a real-time value of how well a row was matched, researchers can make clear methodological choices about which data to include in their analysis or get a powerful method to cluster data points for analysis. On top of better reporting and more options for researchers, the data engineering team is also appending source tags to de-identified data. Every row will have some clue as to its origin. When researchers communicate issues with the de-identified data, we can pin down system behavior to a set of source documents for faster, more collaborative debugging.
How do you use metadata? Join the conversation on Twitter @UTDataResearch.
References
Pickerill, S. (2021, November). What is data enrichment, and how to implement it. Retrieved from SnowPlow: https://snowplowanalytics.com/blog/2021/06/23/what-is-data-enrichment-and-how-to-implement-it/